Analysis Features
New Features
In 2007, SigmaStat’s functions and features were integrated into SigmaPlot starting at version 11. However, on February 1, 2016, SigmaStat 4.0 was released and is now available as a standalone product.
Below are the many improvements to the statistical analysis functions in SigmaStat:
- Principal Components Analysis (PCA) – Principal component analysis is a technique for reducing the complexity of high-dimensional data by approximating the data with fewer dimensions.
- Analysis of Covariance (ANCOVA) – Analysis of Covariance is an extension of ANOVA (Analysis of Variance) obtained by specifying one or more covariates as additional variables in the model.
- Cox Regression – This includes the proportional hazards model with stratification to study the impact of potential risk factors on the survival time of a population. The input data can be categorical.
- One-Sample T-test – Tests the hypothesis that the mean of a population equals a specified value.
- Odds Ratio and Relative Risk tests – Both tests the hypothesis that a treatment has no effect on the rate of occurrence of some specified event in a population. Odds Ratio is used in retrospective studies to determine the treatment effect after the event has been observed. Relative Risk is used in prospective studies where the treatment and control groups have been chosen before the event occurs.
- Shapiro-Wilk Normality test – A more accurate test than Kolmogorov-Smirnov for assessing the normality of sampled data. Used in assumption checking for many statistical tests, but can also be used directly on worksheet data.
- New Result Graph – ANOVA Profile Plots: Used to analyze the main effects and higher-order interactions of factors in a multi-factor ANOVA design by comparing averages of the least square means.
- New Probability Transforms – Thirty four new functions have been added to SigmaStat’s Transform language for calculating probabilities and scores associated with distributions that arise in many fields of study.
- New Interface Change – Nonlinear Regression: An easy to use wizard interface and more detailed reports.
- New Interface Change – Quick Transforms: An easier way of performing computations in the worksheet.
- New Interface Change – New User Interface: Allows the user to work more easily with Excel worksheets.
- Yates correction added to the Mann-Whitney test – Yates correction for continuity, or Yates chi-square test is used when testing for independence in a contingency table when assessing whether two samples of observations come from the same distribution.
- Improved Error Messaging – Improved error messages have added information when assumption checking for ANOVA has failed.
- Deming Regression – Deming regression allows for errors in both X and Y variables – a technique for method comparison where the X data is from one method and the y data the other. The Deming regression method basically extends the normal linear regression, where the X values are considered to be error-free, to the case where both X and Y (both methods) have error. Hypotheses can then be tested, slope different from 1.0 for example, to determine if the methods are the same. For example, it might be used to compare two instruments designed to measure the same substance or to compare two algorithmic methods of detecting tumors in images. The graph compares the two methods to determine if they are different or the same. A report gives statistical results.
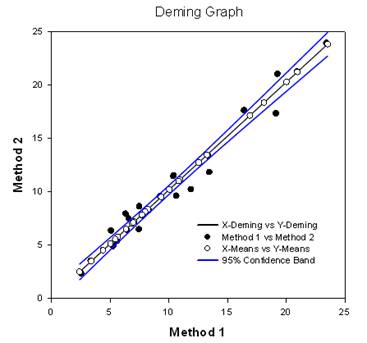
- Akaike Information Criterion (AICc) – The Akaike Information Criterion is now available in nonlinear regression reports. It is a goodness of fit criterion that also accounts for the number of parameters in the equation. It also is valid for non-nested equations that occur, for example, in enzyme kinetics analyses.
- New Probability Functions for Nonlinear Regression – A total of 24 probability functions have been added to the curve fit library. Automatic initial parameter estimate equations have been created for each.
- Nonlinear Regression Weighting – There are now seven different weighting functions built into each nonlinear regression equation (3D are slightly different). These functions are reciprocal y, reciprocal y squared, reciprocal x, reciprocal x squared, reciprocal predicteds, reciprocal predicteds squared and Cauchy. The iteratively reweighted least squares algorithm is used to allow the weights to change during each nonlinear regression iteration.
- Multiple Comparison Test Improvements – Two important improvements have been made. P values for the results of nonparametric ANOVA have been added. These did not exist before. Also, multiple comparison P values were limited to discrete choices (0.05, 0.01, etc.). This limitation no longer exists and any valid P value may be used.
Major New Statistical Tests
Principal Components Analysis (PCA) – Principal component analysis is a technique for reducing the complexity of high-dimensional data by approximating the data with fewer dimensions. Each new dimension is called a principal component and represents a linear combination of the original variables. The first principal component accounts for as much variation in the data as possible. Each subsequent principal component accounts for as much of the remaining variation as possible and is orthogonal to all of the previous principal components.
You can examine principal components to understand the sources of variation in your data. You can also use them in forming predictive models. If most of the variation in your data exists in a low-dimensional subset, you might be able to model your response variable in terms of the principal components. You can use principal components to reduce the number of variables in regression, clustering, and other statistical techniques. The primary goal of Principal Components Analysis is to explain the sources of variability in the data and to represent the data with fewer variables while preserving most of the total variance.
Examples of Principal Components Graphs:
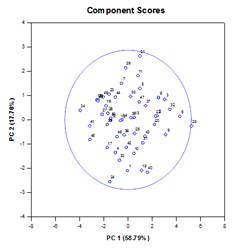
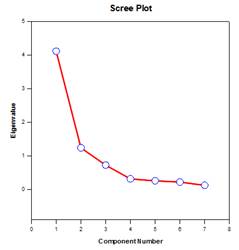
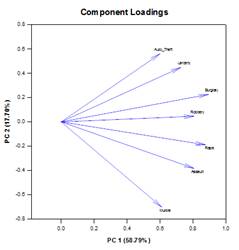
Analysis of Covariance (ANCOVA) – Analysis of Covariance is an extension of ANOVA obtained by specifying one or more covariates as additional variables in the model. If you arrange ANCOVA data in a SigmaPlot worksheet using the indexed data format, one column will represent the factor and one column will represent the dependent variable (the observations) as in an ANOVA design. In addition, you will have one column for each covariate.
Examples of ANCOVA Graphs:
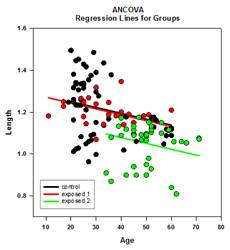
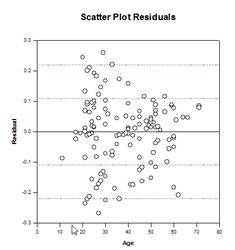
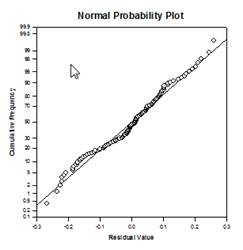
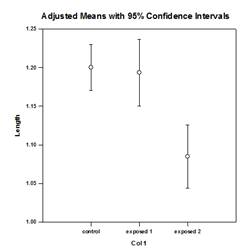
New Statistical Features







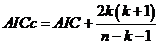
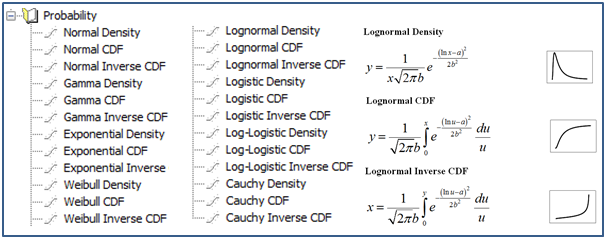
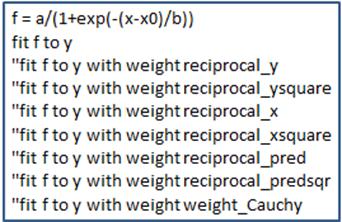